- February 10, 2021
- 12:14 pm
- No Comments
Conversion rate testing has become a cornerstone of innovation and growth. Companies like Google, Netflix, Booking.com are just a few names who fully embrace testing culture – running thousands of experiments at any given time to perfect user experiences. As marketers, we design experiments to optimize websites, validate media strategies, understand our consumers better – you name it. But there’s often an error in the way marketers draw conclusions about test results, and how “conclusive” A/B tests really are.
The A/B testing process typically looks something like this: observe pattern of behavior > hypothesis > test > conclusion. In other words, if the test confirms the theory, then the theory is considered true.
The problem is that our tendency shouldn’t be to seek to confirm something, it should be to seek to find evidence that would disapprove it.
A hypothesis can’t be right unless it can be proven wrong.
– Karl Popper –
Design test in a way that can disprove a theory, not confirm it.
If a hypothesis cannot be proven right or wrong, don’t waste your time and resources to investigate.
This idea can be illustrated with the popular “black swan” parable. In order to prove the assumption “all swans are white,” you would need to find every swan in the world, whereas finding one black swan could confirm the falsity of the statement more effectively than continuously finding white swans and believing the theory to be fact. Same principle applies for marketers – always focus on testing statements that are falsifiable.
A null hypothesis – or opposite hypothesis – can help you think through ways to prove your theory wrong and ensure your theory is in fact falsifiable. In other words, we assume a test variation will have no impact on conversions until proven false. Thus, begins an iterative process of gathering enough experimental evidence for or against the null hypothesis that it’s nearly impossible that it could be true.
How context can change testing outcomes.
Even if you are testing falsifiable claims, it does not mean you can ever really draw permanent conclusions. Why? Because context matters.
In some situations, especially as tests become more complex, it’s worth examining what may result in a different outcome, or ensuring the context you really care about is represented in your testing methods. You shouldn’t ever really consider the losing version to always be the worst of two outcomes, because new evidence and/or context could change that finding. What matters when it comes to context?
Segmentation matters.
Audience segments, traffic sources and even time of day can have major implications on test outcomes. This becomes even more apparent when bulk analysis is involved.
For example – you think your website messaging strategy should be less sales-y and more informative. If you were to run an experiment testing the impact of educational messaging, whether it wins or loses won’t prove that educational messaging is right or wrong in all circumstances. This is especially true if bulk analysis is involved.
Segment analysis might show that educational messaging works very well with high-value prospects and claiming it doesn’t is only true when results are averaged across all users. Or, you might find that educational content improved conversion on desktop but not mobile devices, which could indicate that the layout or length of content isn’t fit for mobile users. Always examine how much of the data you are collecting might influenced by segments that shouldn’t inform best practices.
Ask yourself: Does your test work the same across all segments of users?
Sequence matters.
Results can change dramatically based on the order in which related tests are conducted. Let’s say you observe that 60% of users abandon your checkout process. So, you decide to test your theory that adding a progress bar will increase conversion rate by 10%. Upon testing, you see a 1% drop in conversion rate. One might conclude that you’ve disproved your theory. Sure, the progress bar reduced conversion rates, so it may still be practical to revert to the previous experience for now. But it’s not always so black and white.
Perhaps, the progress bar would have worked, but the checkout process itself has too many steps involved, a vulnerability exposed by the presence of a progress bar. By testing a shorter checkout flow first, you might see dramatically different results from the addition of progress bar functionality.
Ask yourself: Would variation A work better only after implementing variation B?
Time matters.
Tests have a shelf life, especially in digital marketing. The results of today’s test can (and likely will) change tomorrow, a month or a year from now.
For our final example… You believe that offering discounts on the website will increase sales on unsold inventory by 10%. Initial testing shows a disappointing 1% lift in sales – at a net loss. It may be tempting to slam the gavel on the discounts theory as a failure.
Even though your current site traffic isn’t responding to the discounts yet, that may not be the case for future demographics. User preferences shift like the wind when it comes to new experiences and chances are as your company grows, your website traffic will change with it. And with major changes – like offering a discount program – we often see adoption grow over time as users become more accustomed to the change.
Ask yourself: How can the results of this test change over time?
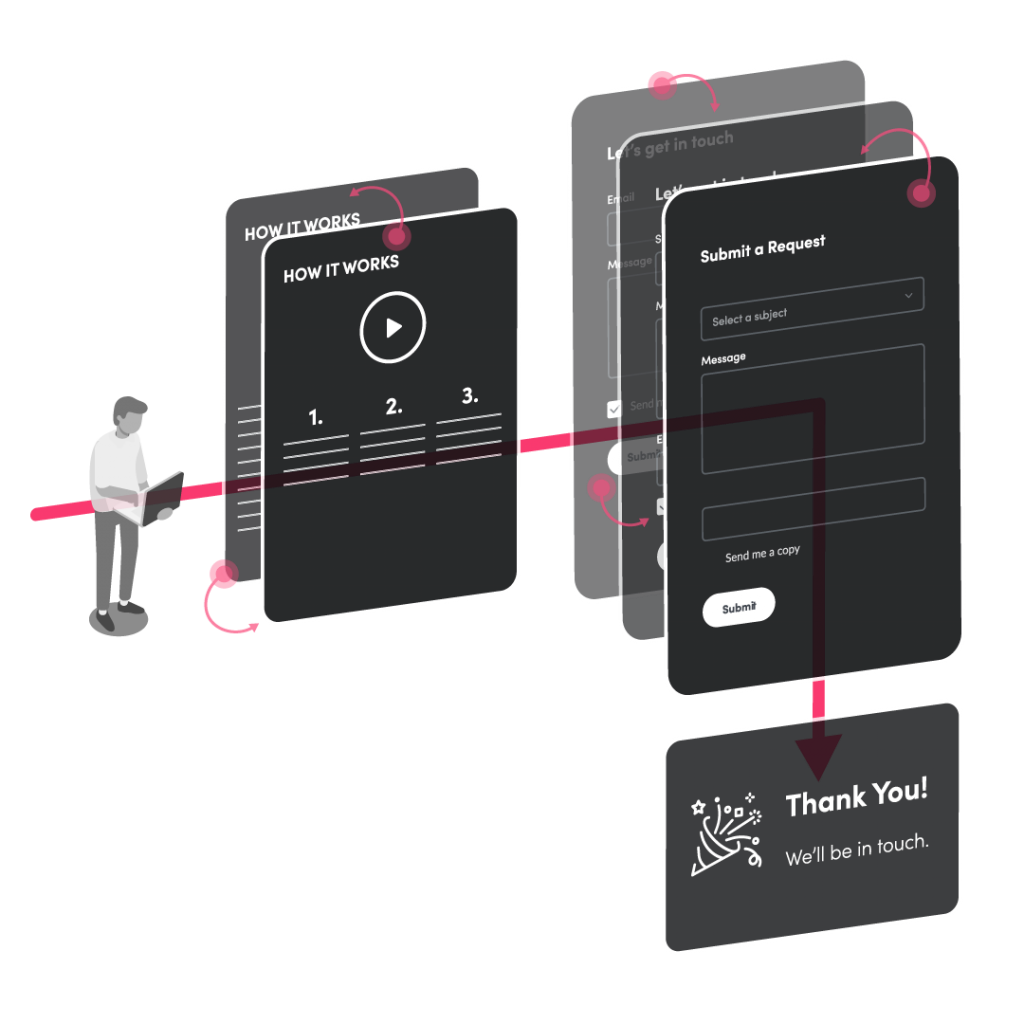
Key takeaways.
- Design your test in a way that would disapprove a theory, not confirm it.
- When you’re designing your test, examine the context the test runs in. How much of the data that you’re capturing is influenced by segments that shouldn’t inform your best practices?
- Unless you could outright disapprove a theory, avoid closing the door on losing variations, and if those variations are immensely important consider follow-up tests where the conditions are different.